Media Mix Modeling: Estimating KPIs During SKAN Limitations
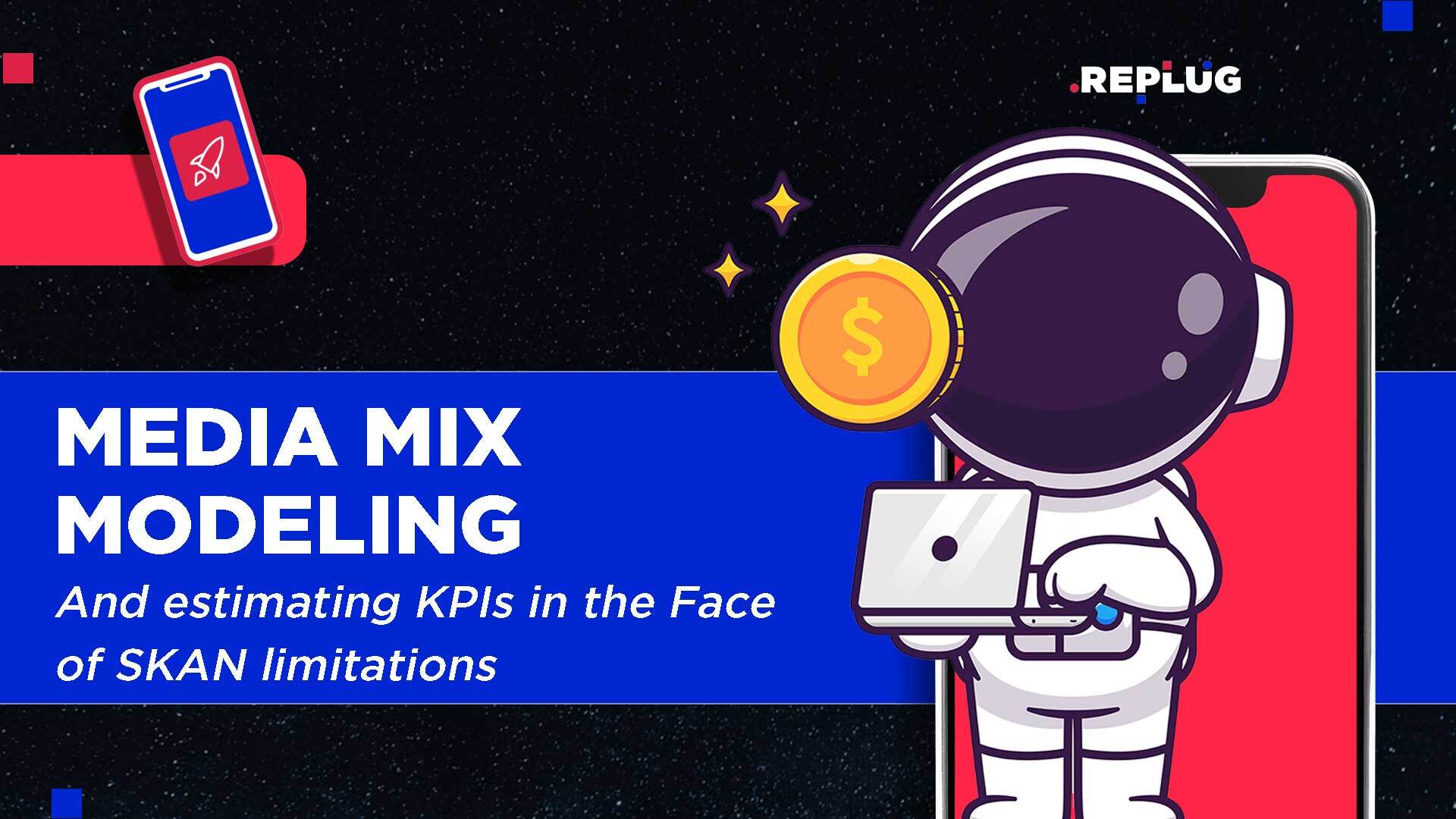
Media Mix Modeling comes in when performance data has proven to be a valuable resource for decision-making, but obtaining insights has become more complex.
Table of Contents
- What is Media Mix Modeling (MMM)?
- What Is the Difference Between MMM and MTA?
- Why Would an Advertiser Use a Mixed Media Approach?
- How Does MMM Work?
- Media Mix: Estimate Post-event Results in SKAN Campaigns
Challenges arise from the restrictions of SKAdNetwork, including a 72-hour window and privacy thresholds, impacting a comprehensive understanding of user conversions (anticipated enhancements with SKAN 4.0 adoption across channels).
Marketers are compelled to navigate these limitations (even in 2024) by making informed estimations for key iOS KPIs, shaping the need for precise decisions in orchestrating effective Media Mix Modeling strategies.
What is Media Mix Modeling (MMM)?
Media Mix Modeling (MMM), also known as marketing mix modeling, is an analytical approach empowering marketers to evaluate the impact of their marketing endeavors, revealing how diverse elements contribute to their primary objective – often geared towards enhancing conversions.
The insights coming from MMM enable marketers to fine-tune their campaigns, considering many factors, from consumer trends to external influencers, in order to make an optimal campaign that drives both engagement and sales.
MMM leverages aggregate data, enabling the evaluation of a broad spectrum of channels implementing both traditional and digital platforms. Furthermore, MMM facilitates the use of external influencers, such as seasonality and promotions, in the analysis, providing marketers with a comprehensive understanding to refine and elevate their strategies effectively.
What Is the Difference Between MMM and MTA?
Regarding app marketing, Media Mix Modeling offers a comprehensive, top-down view of various business facets, aiming to grasp the contribution of each aspect to overall business outcomes.
On the other hand, Multi-Touch Attribution (MTA) takes a meticulous bottom-up approach within the app marketing landscape, striving to precisely measure every consumer interaction with media and attribute each exposure to specific outcomes.
Why Would an Advertiser Use a Mixed Media Approach?
A media mix marketing strategy provides enhanced flexibility and visibility compared to traditional marketing approaches. Integrating various marketing channels into a cohesive media mix enables your business to tap into new markets, enhance brand recognition, and boost the return on investment (ROI) of your marketing efforts.
Moreover, SKAdNetwork’s limitations may result in data gaps and incomplete insights, especially for specific KPIs (Cost Per Event, LTV, e.g.) or segments (retention, user quality). Media Mix Modeling can bridge these gaps by leveraging additional data sources and statistical modeling techniques.
MMM allows marketers to attribute post-install events or KPIs to marketing channels beyond what SKAdNetwork can track. By analyzing historical data and integrating consenting user analysis, estimations can provide insights into specific channels’ effectiveness, even without precise SKAdNetwork measurements.
By incorporating estimations for untracked or underreported channels, MMM can provide a more accurate assessment of the overall performance and help marketers evaluate the effectiveness of their media mix decisions.
How Does MMM Work?
Media Mix Modeling employs statistical analysis, specifically multi-linear regression, to ascertain the correlation between the dependent variable, such as sales or engagements, and the independent variables, such as advertising expenditure across various channels.
The media mix can be calibrated and validated using available SKAdNetwork data as a reference point. By comparing the estimations derived from MMM with the actual SKAdNetwork measurements, marketers can fine-tune and validate the model’s accuracy, ensuring that the estimations align closely with the observed data.
Estimates will vary greatly depending on each case, and calculations should be tailored to specific scenarios or in-app events. That’s where expertise meets data, and all variables should be considered. Estimations should factor in lengths of free trials, cohort analysis with different timeframes, audience segmentation, etc.
Advantages and Disadvantages of Standard Media Mix Marketing
Media Mix Modeling (MMM) offers several advantages and disadvantages for marketers in paid app marketing. Understanding the pros and cons listed below can help marketers make informed decisions about implementing MMM in their strategies.
Advantages of Media Mix Modeling
- Data-driven insights: it provides valuable insights into the effectiveness of different media channels by analyzing historical data. Marketers can make data-driven decisions about budget allocation and media planning, optimizing their strategies for better results.
- ROI optimization: media mix modeling helps marketers identify the media channels that generate the highest return on investment (ROI). Marketers can maximize their marketing efforts and achieve better ROI by allocating resources to the most effective channels.
- Synergy analysis: it enables marketers to evaluate the synergistic effects of combining different media channels. Understanding how channels work together allows for more effective integrated marketing campaigns, leveraging the strengths of each channel to amplify overall impact.
Disadvantages of Media Mix
- Complexity and expertise: implementing MMM requires statistical modeling expertise and a deep understanding of data analysis. It can be a complex process that requires skilled professionals and adequate resources.
- Time and resources: MMM requires substantial data collection and analysis. Gathering the necessary data, processing it, and running the models can be time-consuming and resource-intensive.
- Limited granularity: media mix modeling operates on aggregated data, which can limit the granularity of insights. Individual-level data may not be available or feasible to analyze, potentially missing out on specific nuances or individual channel effects.
- Lag time: it relies on historical data, which means there may be a lag between the actual marketing efforts and the availability of insights. Real-time adjustments may not be possible, impacting the agility of marketing campaigns.
The Four Standard Stages of an MMM Process
The four steps of an effective, standard MMM procedure are as follows:
- Collection of data: here, you must concentrate on gathering first-party data in order to provide a more precise picture of user reactions and behavior in regard to your marketing strategy. Collect data on prior marketing, non-marketing, and outside factors. Examples include user engagement, target audience demographics, and ad spending.
- Modeling: MMM works better with online channels. Traditional techniques, such as print and broadcasts, are more difficult to track. As an app marketer, you may use multi-linear regression to calculate ROI and provide reliable, credible decision-making insights. Pick a dependent variable like revenue or app downloads to build an MMM model. Determine the independent variables.
- Insights and data analysis: you’ll utilize the model from phase 2 to identify and analyze insights about your marketing initiatives in this stage. Assess each channel’s impact on the business outcomes and reliable metrics you established. Based on income or user interaction, you can rank your marketing initiatives. Media effectiveness, efficiency, and ROI can be measured for each campaign.
- Optimization: here, you adjust your marketing mix for future campaigns based on phase three results. Try replicating multiple marketing situations, targeting different groups, or adjusting ad spend levels to determine the best combination of techniques for achieving your revenue targets faster.
Media Mix: Estimate Post-event Results in SKAN Campaigns
Below is a general scenario that we use. Specific cases may require specific adjustments to the example below. The idea here is to incorporate the “CAMPAIGN A” consenting user conversion rate (CR%) into “CAMPAIGN A” SKAN numbers.
In cases where the privacy threshold is not passed:
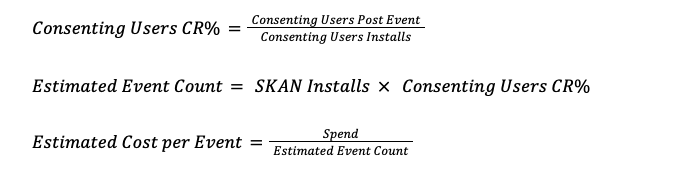
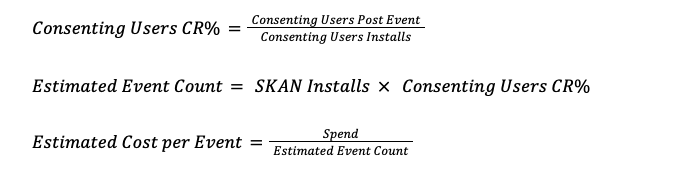
In cases where the privacy threshold is passed, but we want a CPA for a longer cohort period (e.g., 14 days):
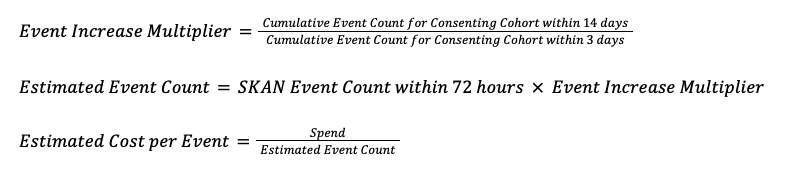
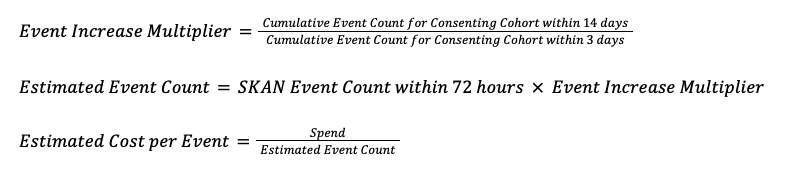
Note: In cases where there is insufficient consenting user data, we consider organic users as well.
Conclusion
Marketers should approach Media Mix Modeling (MMM) and estimations as iterative processes, constantly updating and refining their models as new data becomes available. This approach allows them to adapt to changing market dynamics and consumer behavior, ensuring their media strategies remain optimized over time.
With the inclusion of SKAN in a marketer’s reality, it has become essential to acknowledge its limitations and the need for data. In order to overcome these limitations, marketers should employ educated estimations to supplement the available data in 2024.
Need help measuring the true impact of a campaign? Our team of experts will help you measure, manage, and analyze marketing performance data to understand the effectiveness and improve ROI.
Originally published on July 7, 2023. Updated on October 23, 2023.